AI & Traditional SAAS Businesses
I was talking to a freind (@dillonrgardner) about this paper a16z about how software margins and how AI plays will be part of the eco system. '
A few thoughts: I buy the basic premise: AI/ML business don't scale as well as SAAS as a general rule. Things that reduce margins and scale:
- Often, new customers bring in new corner cases which requires new training data and expensive training pipelines.
- Training Data is a messy ball of wax because you need to a) generate it b) build infrastructure to manager it c) label it in many cases
- For a lot of applications (eg. vision, more advanced NLP) there are realistically a small number of players that will give you SOTA performance. Meaning the FAANGS of the world have a natural advantage over smaller players. Take for example any recent CNN architecture is probably at least $1 million in cloud cost just to design the model (see section 5 of a recent example and this for more context).
- NB Privacy laws and requirements can substantially affect the above costs and margins.
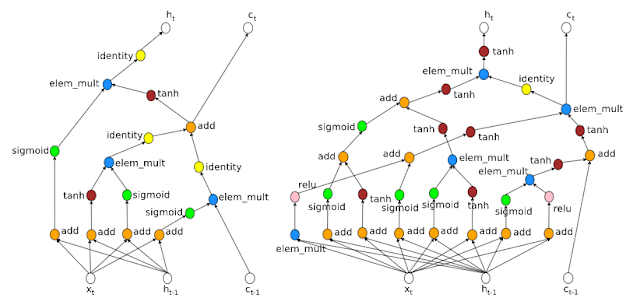
One thing that I think is less important than what the article describes is the issue of inference and Moore's law effects: even in vision applications there are not that many DL models that are bottlenecked by processing power alone. The only exception to this is in a safety critical space like driving where you need every. ounce. of. accuracy.
AI and software are not that different in one key aspect:
FTA:
Handling this huge state space tends to be an ongoing chore. Since the range of possible input values is so large, each new customer deployment is likely to generate data that has never been seen before.
This is a key consideration when thinking about scaling an AI business: what applies for regular software applies ML: complexity kills and you are well-advised to reformulate your problem domain to severely limit possibilities of corner cases as much as possible.